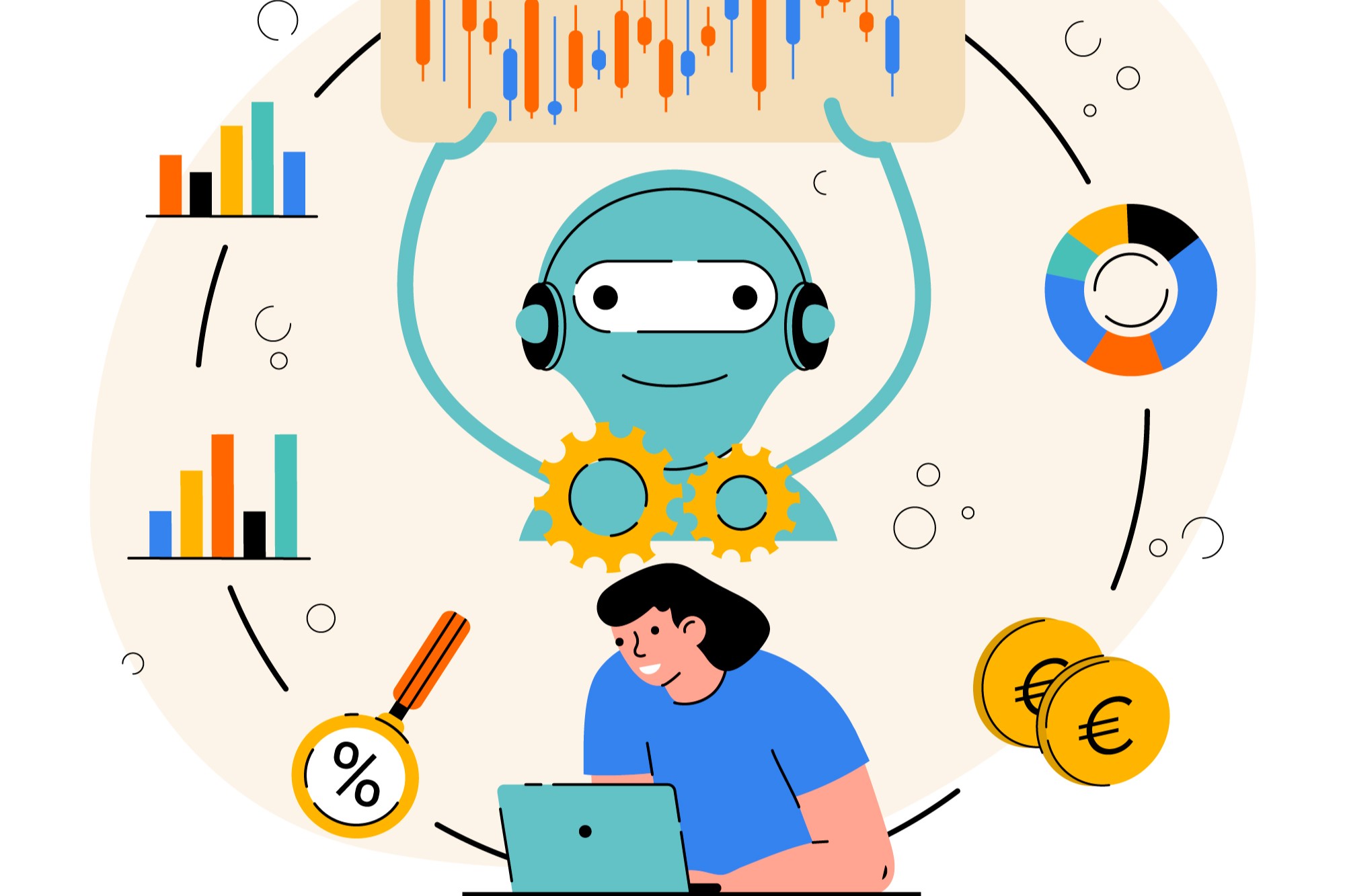
Unveiling the intricacies of AI trading strategies, this article explores the diverse methodologies employed by algorithms to navigate complex market conditions. From trend analysis to sentiment detection, AI-driven strategies offer a systematic approach to achieving consistent returns. Gain insights into the evolution of these strategies and their impact on modern trading practices.
Importance of AI in Enhancing Trading Strategies
AI has revolutionized the field of trading by providing advanced tools and techniques that significantly improve the efficiency and accuracy of trading strategies. By leveraging vast amounts of data and powerful computational capabilities, AI systems can analyze market trends, predict price movements, and execute trades at speeds and accuracies far beyond human capabilities. This allows traders to identify and exploit market opportunities more effectively, leading to higher profitability and reduced risk.
Furthermore, AI-driven trading strategies offer a level of adaptability and flexibility that traditional methods lack. Machine learning algorithms, for instance, can continuously learn from new data, enabling them to adjust to changing market conditions in real-time. This dynamic approach not only enhances the precision of trading decisions but also helps in mitigating potential losses by quickly responding to unexpected market shifts. As a result, AI is becoming an indispensable tool for traders seeking to maintain a competitive edge in the rapidly evolving financial markets.
Types of AI Trading Strategies
AI trading strategies encompass various methodologies that leverage advanced algorithms and computational techniques to navigate financial markets. Here are some key types:
Machine Learning Algorithms
- Supervised Learning: Utilizes labeled historical data to train models for predicting future market movements.
- Unsupervised Learning: Analyzes unlabeled data to identify patterns and relationships within market data, useful for clustering and anomaly detection.
Natural Language Processing (NLP) in Trading
- Sentiment Analysis: Processes news articles, social media, and other textual data to gauge market sentiment and predict market reactions.
- Text Mining: Extracts actionable insights from unstructured textual data relevant to trading decisions.
Reinforcement Learning in Trading
- Policy Gradient Methods: Trains agents to maximize rewards by interacting with simulated or historical market data.
- Q-Learning: Utilizes value functions to learn optimal trading strategies based on rewards and penalties received from simulated trading environments.
These strategies illustrate how AI techniques can be tailored to different aspects of trading, from predictive analytics to real-time decision-making, enhancing overall trading performance and profitability.
Natural Language Processing (NLP) in Trading
Natural Language Processing (NLP) has emerged as a powerful tool in trading, allowing traders to analyze and interpret vast amounts of textual data that can influence market movements. By processing news articles, social media posts, financial reports, and other text sources, NLP can assess the sentiment and tone of the information, providing insights into market trends and potential price movements. For example, positive or negative sentiment extracted from a major company’s earnings report or a key economic indicator can significantly impact stock prices, and NLP enables traders to capitalize on these insights promptly.
Additionally, NLP facilitates the extraction of relevant information from unstructured data, enabling traders to stay informed about market conditions and events in real-time. Text mining techniques help identify significant events, such as mergers and acquisitions, regulatory changes, or geopolitical developments, which might affect market behavior. By integrating NLP into their trading strategies, traders can make more informed decisions based on comprehensive data analysis, thereby improving their ability to anticipate market changes and react swiftly to emerging opportunities. This capability is particularly valuable in high-frequency trading environments where speed and accuracy are critical.
Reinforcement Learning in Trading
Reinforcement Learning (RL) represents a cutting-edge approach in AI trading strategies, wherein models learn optimal trading policies through trial and error. Unlike traditional supervised learning, which relies on historical data with known outcomes, RL involves agents that interact with a trading environment, receiving rewards or penalties based on their actions. This continuous feedback loop enables the agents to refine their strategies over time, aiming to maximize cumulative rewards.
Key Components and Methods
- Policy Gradient Methods: These methods optimize the trading strategy directly by adjusting the policy parameters in the direction that increases expected rewards. They are particularly effective in environments with continuous action spaces, making them suitable for trading scenarios that require nuanced decision-making.
- Q-Learning: A value-based method where the agent learns a value function that estimates the expected rewards for taking a given action in a specific state. This method helps in identifying the optimal action-value function, guiding the agent towards actions that maximize long-term rewards. Variants like Deep Q-Networks (DQN) leverage neural networks to handle large state-action spaces, enhancing their applicability in complex trading environments.
Applications in Trading
- Market Making: RL agents can be used to develop market-making strategies, where the goal is to provide liquidity by continuously buying and selling securities. The agents learn to balance inventory and manage the bid-ask spread to optimize profitability while minimizing risk.
- Portfolio Management: RL can assist in dynamic portfolio management by continuously adjusting asset allocations in response to changing market conditions. The agent learns to optimize the trade-off between risk and return, adapting to market trends and volatility.
By leveraging reinforcement learning, traders can develop adaptive and robust strategies that respond effectively to the ever-changing dynamics of financial markets. This approach not only enhances decision-making but also provides a framework for continuously improving trading performance through experiential learning.
Quantitative Trading Strategies
Quantitative trading strategies leverage mathematical models and statistical techniques to identify and exploit trading opportunities. These strategies rely on vast amounts of historical data and computational power to generate trading signals and make data-driven decisions.
Strategy Type | Traditional Quantitative Strategies | AI-Driven Quantitative Strategies |
Approach | Relies on predefined mathematical models and rules | Utilizes machine learning algorithms and data-driven models |
Adaptability | Limited adaptability to changing market conditions | Highly adaptable, continuously learning from new data |
Complexity | Relatively straightforward, based on established theories | More complex, involving advanced computational techniques |
Types of Quantitative Trading Strategies
- Statistical Arbitrage: This strategy involves identifying price inefficiencies between related financial instruments and executing trades to profit from the convergence of prices. AI enhances this approach by improving the accuracy of statistical models and enabling real-time analysis of vast data sets, leading to more precise and timely arbitrage opportunities.
- Algorithmic Trading: Algorithmic trading strategies execute pre-programmed instructions for trading at high speeds and volumes. AI-driven algorithms can process and analyze market data in real-time, making split-second decisions that maximize trading efficiency and profitability. This approach is particularly effective in high-frequency trading environments where speed is crucial.
- Factor-Based Investing: This strategy involves selecting securities based on specific characteristics, or “factors,” such as value, momentum, or volatility. AI can identify and optimize these factors more effectively by analyzing large and complex data sets, uncovering hidden patterns and relationships that traditional methods might miss. This results in more robust and adaptive factor models that can better navigate diverse market conditions.
By integrating AI into quantitative trading strategies, traders can enhance their ability to analyze data, identify trading opportunities, and execute trades more efficiently. This results in improved performance and a greater ability to adapt to the dynamic nature of financial markets.
Leave a Reply