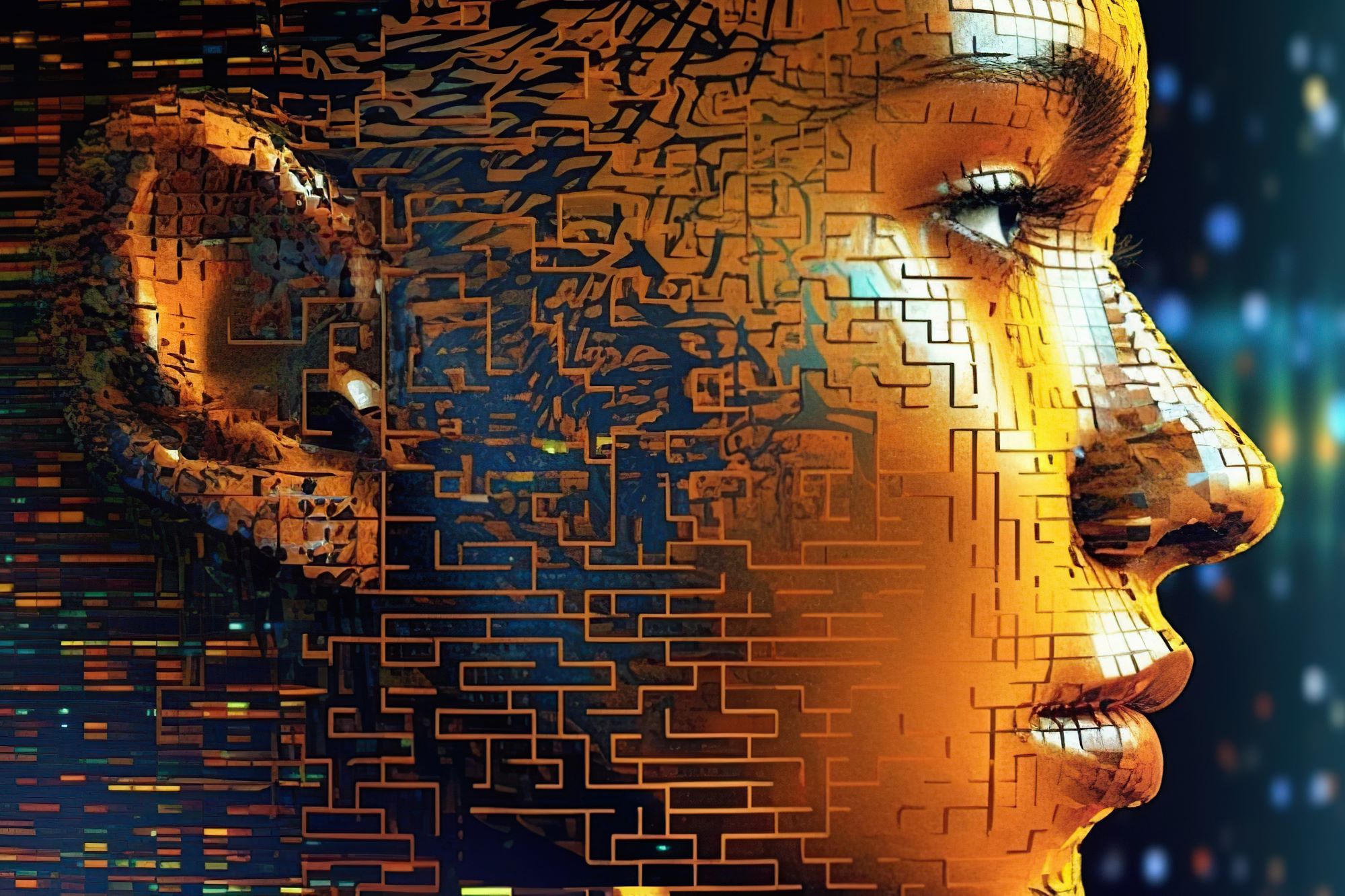
As AI assumes a greater role in trading decisions, ethical considerations emerge regarding fairness, transparency, and accountability. This article examines the ethical implications of AI in trading, addressing concerns such as algorithmic bias, market manipulation, and regulatory challenges. Explore the ethical dilemmas shaping the future of AI-driven trading.
Transparency and Accountability
Transparency in AI algorithms used for trading is crucial for maintaining trust and integrity in financial markets. When algorithms operate as black boxes, their decision-making processes become opaque, making it difficult for stakeholders to understand how specific trading decisions are made. This lack of transparency can lead to mistrust among market participants and regulators, as well as potential misuse or unintended consequences of AI-driven trading strategies. Ensuring that AI models are interpretable and their operations are clearly documented can help build confidence and ensure that they are being used ethically and effectively.
Accountability is another critical aspect, as it pertains to identifying who is responsible for the outcomes generated by AI trading systems. When AI systems make trading decisions that result in significant financial losses or market disruptions, determining liability can be challenging. Regulatory bodies must establish clear guidelines and frameworks to ensure that companies deploying AI in trading are held accountable for their systems’ actions. This includes implementing robust monitoring mechanisms and requiring firms to maintain detailed records of their AI operations to facilitate auditing and oversight.
Fairness and Bias
Fairness in AI trading systems is essential to ensure that all market participants have equal opportunities and are not unfairly disadvantaged by algorithmic decisions. Bias in AI models can lead to discriminatory practices, where certain groups or individuals are systematically favored or disfavored. This can occur due to biased training data or inherent biases in the model design. Addressing these biases is crucial to maintain a fair and equitable trading environment.
Sources of Bias in AI Trading Systems:
- Historical Data Bias: Training data that reflects historical inequalities or market behaviors can perpetuate those biases in AI models.
- Algorithmic Bias: The design and structure of the AI model itself can introduce biases, especially if it prioritizes certain types of trades or data.
- Human Bias: The biases of developers and data scientists can inadvertently influence the AI models they create.
Strategies to Mitigate Bias:
- Diverse Training Data: Ensure that the training data is representative of various market conditions and participants to minimize historical biases.
- Bias Detection Tools: Implement tools and techniques to detect and measure bias in AI models, allowing for ongoing monitoring and adjustment.
- Regular Audits: Conduct regular audits of AI trading systems to identify and address any emerging biases.
- Inclusive Development Teams: Promote diversity within development teams to reduce the risk of human biases influencing AI model design.
By actively addressing and mitigating bias in AI trading systems, the industry can move towards more fair and equitable trading practices that benefit all market participants.
Market Manipulation
Aspect of Market Manipulation | Examples | Regulatory Response |
False Information | Spreading false rumors to influence stock prices. | Imposing fines and penalties on perpetrators. |
Pump and Dump Schemes | Artificially inflating the price of a security. | Monitoring and surveillance to detect unusual trading. |
Spoofing | Placing large orders to manipulate market perception. | Implementing trading rules to prevent spoofing tactics. |
- False Information
- Spreading false rumors or misinformation to manipulate stock prices.
- Regulatory response involves imposing fines and penalties on those found guilty of disseminating false information.
- Pump and Dump Schemes
- Artificially inflating the price of a security through coordinated buying.
- Regulatory bodies monitor trading activities and employ surveillance mechanisms to detect unusual trading patterns associated with pump and dump schemes.
- Spoofing
- Placing large orders with no intention of execution to manipulate market perception.
- Regulators implement trading rules and algorithms designed to detect and prevent spoofing tactics, ensuring market fairness and integrity.
Addressing market manipulation through regulatory measures is crucial to maintaining fair and transparent financial markets, where AI can play a role in both detecting and potentially perpetrating such activities.
Privacy Concerns
The use of personal and sensitive data in AI trading systems raises significant privacy concerns. AI algorithms often rely on vast amounts of data to make accurate predictions and decisions, including information about individual traders, their trading behaviors, and other financial activities. This data collection can lead to potential breaches of privacy if not handled properly. Unauthorized access or misuse of this data can result in significant harm to individuals, including identity theft, financial loss, and erosion of trust in financial institutions.
Balancing data utility and privacy is a critical challenge in the development and deployment of AI trading applications. While comprehensive data is essential for the effectiveness of AI models, safeguarding this data is equally important. Implementing strong data protection measures, such as encryption, anonymization, and strict access controls, can help mitigate privacy risks. Additionally, adhering to data privacy regulations and ensuring transparency about data usage practices can foster trust and protect the rights of individuals whose data is being used.
Job Displacement
Job displacement due to the automation of trading processes by AI technologies poses significant ethical and economic challenges. As AI becomes more prevalent in financial markets, certain roles traditionally performed by humans are increasingly being automated, leading to potential job losses and shifts in employment dynamics.
Impact on Employment in the Trading Sector:
- Trading Floor Roles: Traditional trading roles, such as floor traders and market makers, are increasingly being replaced by automated trading algorithms.
- Back Office Operations: Administrative roles involved in trade processing and settlement are being streamlined or automated.
- Analyst and Research Positions: AI tools are augmenting or replacing roles that involve data analysis and market research.
Ethical Implications of Job Displacement:
- Unemployment: Displaced workers may face challenges in finding new employment opportunities that match their skills and experience.
- Income Inequality: Automation in trading could exacerbate income disparities if new job opportunities created by AI adoption are not accessible to displaced workers.
- Training and Transition Support: Ethical considerations include providing retraining programs and support for displaced workers to transition to new roles within or outside the financial sector.
Managing the ethical implications of job displacement in AI-driven trading requires proactive measures from both industry leaders and policymakers to ensure that technological advancements benefit society as a whole while mitigating the negative impacts on individuals and communities affected by automation.
Leave a Reply