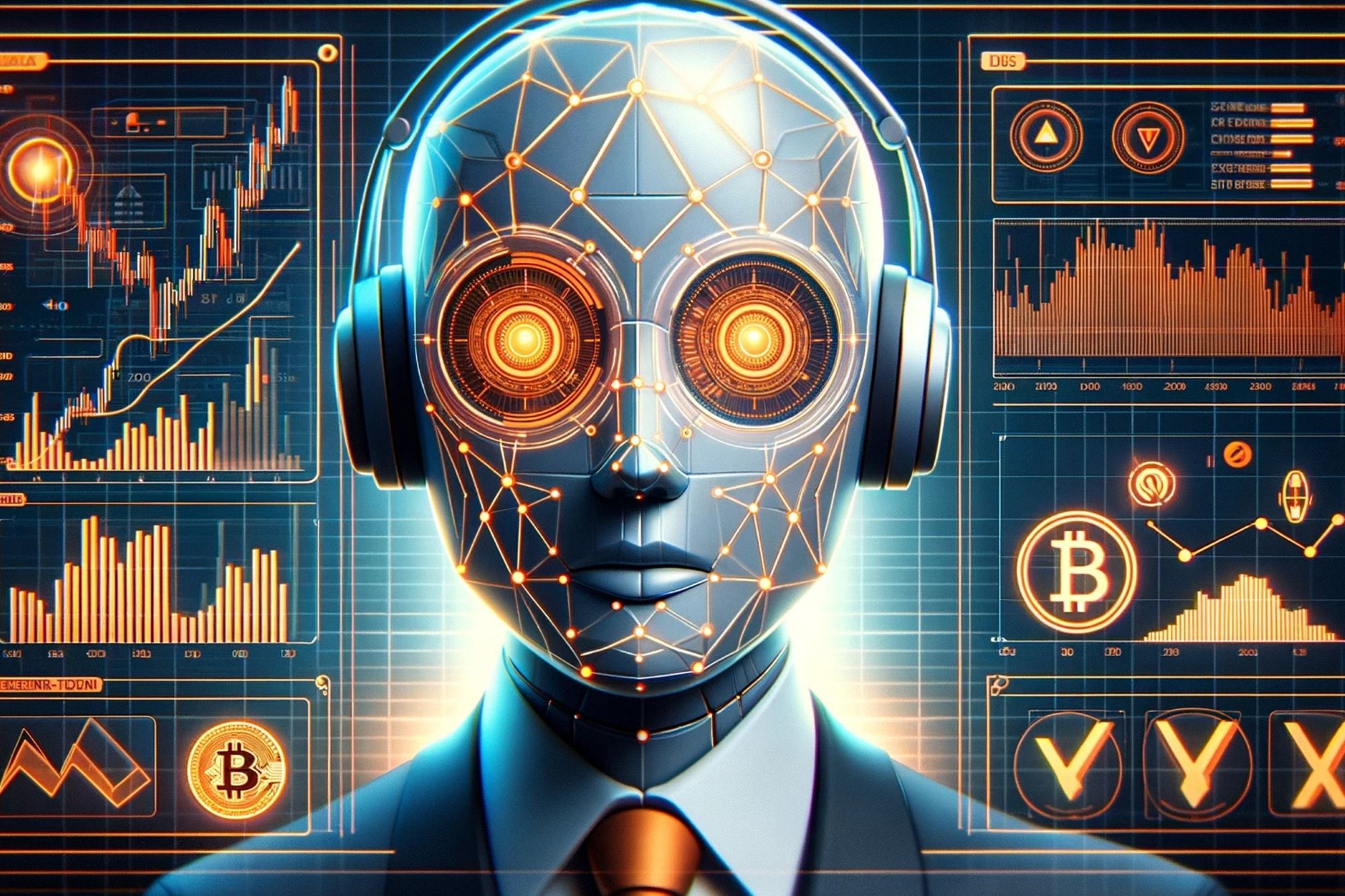
Beyond trading, ai trading bot influence extends into various facets of finance, revolutionizing risk management, customer service, and regulatory compliance. This article explores the broader implications of AI in financial services, highlighting innovative applications and future trends. Discover how AI is reshaping the entire financial landscape beyond trading activities.
Risk Management and Fraud Detection
In the realm of finance, AI plays a crucial role in enhancing risk management and fraud detection processes. Traditionally, these tasks have relied heavily on historical data analysis and rule-based systems. However, AI introduces a paradigm shift by leveraging machine learning algorithms to detect patterns and anomalies in real-time data streams. For instance, machine learning models can analyze vast amounts of transactional data to identify unusual behaviors that may indicate fraudulent activities. These models continuously learn from new data, improving their accuracy over time and adapting to evolving fraud tactics.
Moreover, AI-powered risk management systems can assess risk factors more comprehensively than traditional methods. By integrating diverse data sources and applying advanced analytics, AI enables financial institutions to assess creditworthiness more accurately and manage portfolio risks effectively. This proactive approach not only minimizes financial losses but also enhances customer trust by ensuring robust security measures are in place. As AI continues to evolve, its application in risk management and fraud detection is expected to become even more sophisticated, providing financial institutions with powerful tools to safeguard assets and maintain regulatory compliance.
Customer Service and Personalization
AI has revolutionized customer service in the finance sector by introducing advanced automation and personalization capabilities. Here’s how:
- Chatbots and Virtual Assistants: AI-powered chatbots and virtual assistants handle customer queries and requests in real-time, improving response times and availability. These bots can provide 24/7 support, handle routine inquiries, and escalate complex issues to human agents when necessary.
- Personalized Recommendations: Utilizing machine learning algorithms, financial institutions can analyze customer data to offer personalized product recommendations and financial advice. This not only enhances customer satisfaction but also increases the likelihood of cross-selling relevant products.
- Behavioral Analysis: AI algorithms analyze customer interactions and behaviors across digital platforms to anticipate needs and preferences. By understanding individual customer preferences, financial institutions can tailor their services and communication strategies effectively.
- Sentiment Analysis: Natural language processing (NLP) techniques enable sentiment analysis of customer feedback and social media interactions. Financial institutions can gauge customer satisfaction levels in real-time and address concerns promptly, thereby improving overall service quality.
AI-driven customer service not only improves operational efficiency but also enhances the overall customer experience, making financial interactions more seamless and personalized. As AI technologies continue to evolve, the potential for deeper customer insights and more sophisticated service offerings grows, further transforming the finance industry.
Algorithmic Credit Scoring
Traditional Credit Scoring | Algorithmic Credit Scoring | Advantages of Algorithmic Scoring |
Relies on historical data and fixed rules | Uses machine learning to analyze vast datasets and real-time information | Improved accuracy in credit assessment |
May not adapt quickly to changing economic conditions or borrower behaviors | Adapts dynamically to new data and market trends | Faster decision-making and reduced risk |
Often based on a limited set of variables | Incorporates a wide range of factors, including alternative data sources | Enhances fairness and inclusivity in lending practices |
- Machine Learning Models: Algorithmic credit scoring employs machine learning models such as decision trees, neural networks, and ensemble methods to analyze borrower data comprehensively. These models can identify complex patterns and correlations that traditional scoring methods might overlook.
- Integration of Alternative Data: Beyond traditional credit bureau data, algorithmic scoring integrates alternative data sources such as payment histories, social media behavior, and even educational background. This provides a more holistic view of a borrower’s creditworthiness, especially beneficial for individuals with limited credit histories.
- Real-time Decision Making: By processing data in real-time, algorithmic scoring enables financial institutions to make faster and more informed credit decisions. This agility is crucial in responding to market dynamics and customer expectations promptly.
- Continuous Learning and Adaptation: Machine learning algorithms continuously learn from new data inputs, improving their predictive accuracy over time. This adaptive capability allows lenders to refine risk assessments and adjust lending criteria dynamically.
Algorithmic credit scoring represents a significant advancement in the finance industry, promising more accurate, fair, and efficient credit evaluation processes. As regulatory frameworks evolve to accommodate these technologies, their adoption is likely to accelerate, reshaping how financial institutions assess credit risk and serve borrowers globally.
Market Research and Predictive Analytics
In finance, market research and predictive analytics powered by AI have transformed how institutions anticipate market trends, consumer behavior, and investment opportunities. AI algorithms can analyze vast amounts of data from diverse sources, including financial reports, social media trends, and macroeconomic indicators. This capability enables financial analysts and strategists to uncover hidden patterns and correlations that traditional methods may miss.
Moreover, predictive analytics leverages historical data to forecast future market movements with greater accuracy. Machine learning models can detect subtle signals and trends, helping investors and decision-makers make informed choices regarding asset allocation, portfolio management, and risk mitigation strategies. This proactive approach not only enhances investment outcomes but also supports strategic decision-making by providing actionable insights into market dynamics.
As AI technologies continue to evolve, the scope and precision of predictive analytics in finance are expected to expand further. Institutions that harness these capabilities effectively gain a competitive edge by staying ahead of market shifts and capitalizing on emerging opportunities in a dynamic global economy.
Operational Efficiency and Automation
Operational efficiency and automation in finance have been significantly enhanced by AI technologies, revolutionizing traditional processes and workflows. Here’s how AI contributes:
- Process Automation: AI-powered robotic process automation (RPA) handles repetitive tasks such as data entry, transaction processing, and report generation. This reduces human error, speeds up operations, and frees up human resources for more strategic activities.
- Risk Management: AI algorithms analyze vast datasets in real-time to identify potential risks and anomalies. This proactive risk management approach helps financial institutions mitigate operational risks and comply with regulatory requirements more effectively.
- Cost Reduction: By automating routine tasks and optimizing resource allocation, AI reduces operational costs significantly. This includes savings from streamlined workflows, reduced manual interventions, and better resource utilization.
- Customer Service Enhancement: AI-driven chatbots and virtual assistants provide round-the-clock customer support, handling inquiries, and resolving issues promptly. This enhances customer satisfaction and loyalty while reducing service delivery costs.
- Predictive Maintenance: In operational settings, AI can predict maintenance needs for equipment and infrastructure based on data analysis and historical patterns. This predictive capability minimizes downtime, optimizes asset utilization, and lowers maintenance costs.
AI’s role in enhancing operational efficiency and automation continues to grow, offering financial institutions opportunities to streamline processes, reduce costs, and improve service delivery. As AI technologies advance, the potential for further efficiencies and innovations in operational management becomes increasingly promising.
Leave a Reply