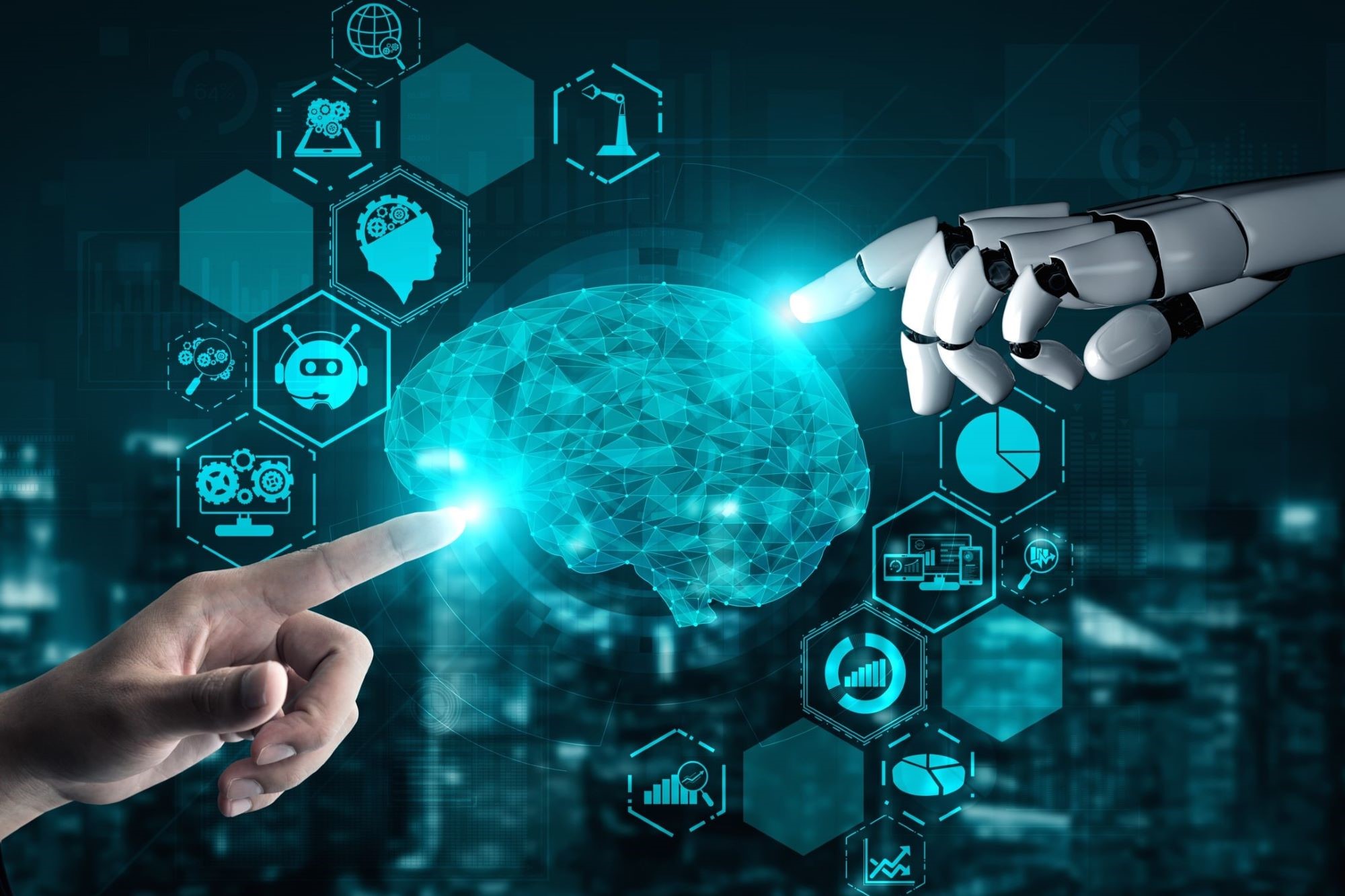
Amidst the hype surrounding AI in trading, separating myths from reality is crucial for informed decision-making. This article debunks common misconceptions and explores the actual capabilities and limitations of AI in financial markets. By examining real-world examples and expert opinions, uncover the truth behind AI’s impact on trading outcomes.
Myth 1: AI Always Beats Human Traders
Many believe that artificial intelligence (AI) consistently outperforms human traders due to its computational power and ability to process vast amounts of data. This belief stems from notable instances where AI-driven algorithms have made impressive predictions or generated substantial returns in financial markets. However, the reality is more nuanced. While AI excels in tasks like pattern recognition and executing trades at high speeds, it is not infallible.
Market conditions can change rapidly, rendering historical data less relevant and challenging AI models to adapt quickly. Moreover, human traders bring intuition, creativity, and the ability to interpret complex contextual factors that AI currently struggles to replicate consistently. In practice, the most successful trading strategies often integrate AI as a tool alongside human expertise, leveraging each’s strengths to navigate the dynamic landscape of financial markets effectively.
Reality 1: AI as a Tool, Not a Magic Bullet
AI serves as a powerful tool in trading, enhancing decision-making processes with its ability to analyze vast amounts of data quickly and detect patterns that may not be obvious to human traders. However, it is crucial to recognize that AI operates based on algorithms and historical data, which may not always capture emerging market trends or unexpected events. Human oversight remains essential to interpret AI outputs, validate conclusions, and adjust strategies based on qualitative insights and contextual understanding.
Complementing Human Expertise
Rather than replacing human traders, AI complements their skills by automating routine tasks, minimizing emotional biases, and executing trades with precision and speed. Human traders contribute crucial elements such as intuition, strategic thinking, and the ability to assess qualitative factors that AI may struggle to comprehend fully. Successful trading strategies often integrate AI as a supportive tool, allowing human traders to focus on higher-level decision-making and adaptability in response to evolving market dynamics.
Myth 2: AI Eliminates the Need for Human Traders
Many perceive AI as a disruptive force that will eventually replace human traders entirely. This belief arises from the notion that AI can execute trades faster, with more precision, and without the emotional biases that human traders often face. However, the reality is far more complex:
- Complementary Roles: AI serves as a powerful tool for data analysis, pattern recognition, and executing trades at high speeds. It can automate routine tasks and handle large volumes of data more efficiently than humans.
- Human Judgment and Intuition: Despite AI’s capabilities, human traders bring critical judgment, intuition, and adaptability to the trading process. They can interpret nuanced market signals, factor in qualitative data, and make strategic decisions that consider broader economic trends and geopolitical events.
- Complex Decision-Making: Trading often involves navigating unpredictable market dynamics, unexpected events, and regulatory changes. Human traders excel in scenarios where context, judgment, and nuanced understanding play crucial roles, areas where AI may struggle.
In practice, successful trading strategies often leverage AI as a tool to enhance decision-making rather than replace human involvement entirely. The symbiotic relationship between AI and human traders highlights the importance of collaboration to achieve optimal outcomes in the evolving landscape of financial markets.
Reality 2: Human Expertise in AI-Driven Trading
To illustrate the symbiotic relationship between AI and human expertise in trading, consider the following breakdown:
Aspect | AI Contribution | Human Expertise Contribution |
Data Analysis | Processes vast amounts of data quickly and accurately. | Provides context, interprets qualitative data. |
Execution | Executes trades rapidly and efficiently based on data. | Makes judgment calls, adjusts strategy in real-time. |
Pattern Recognition | Identifies complex patterns and trends. | Recognizes anomalies, adapts strategies accordingly. |
- Enhanced Data Analysis: AI excels in processing and analyzing large datasets at high speeds, providing insights into market trends and correlations that may not be immediately apparent to human traders.
- Efficient Execution: AI can execute trades swiftly and with minimal error, leveraging predefined algorithms to capitalize on opportunities or manage risks effectively.
- Pattern Recognition: AI algorithms are adept at identifying intricate patterns in market data, facilitating predictive analytics and automated decision-making.
In parallel,
- Contextual Interpretation: Human traders bring nuanced understanding and qualitative analysis to the table, interpreting market events, news, and geopolitical factors that AI may struggle to contextualize accurately.
- Real-time Adjustment: Human traders can adapt trading strategies dynamically in response to sudden market shifts or unexpected events, leveraging their intuition and experience to make informed decisions.
- Strategic Decision-making: Human judgment remains crucial in making strategic decisions that consider long-term market trends, regulatory changes, and broader economic factors that influence trading outcomes.
This collaborative approach underscores the importance of integrating AI capabilities with human expertise to optimize trading strategies effectively in today’s dynamic financial markets.
Myth 3: AI Trading is Always Profitable
There’s a prevalent misconception that AI-powered trading strategies guarantee consistent profitability due to their advanced algorithms and data processing capabilities. However, the reality paints a more nuanced picture:
- Market Volatility: AI trading systems may struggle during periods of high volatility when market conditions deviate significantly from historical data patterns.
- Overfitting Risks: Optimizing AI models based on past data can lead to overfitting, where the model performs well in historical simulations but fails to generalize to new market conditions.
- Complexity of Financial Markets: Financial markets are influenced by numerous unpredictable factors such as geopolitical events, economic policy changes, and natural disasters, which AI models may struggle to incorporate effectively.
In practice,
- Risk Management Challenges: AI trading systems may fail to account for unforeseen risks or black swan events, potentially leading to significant losses.
- Liquidity Issues: AI algorithms may encounter difficulties in executing trades smoothly in illiquid markets or during rapid price movements.
- Regulatory Compliance: Compliance with evolving regulatory frameworks poses challenges for AI trading systems, requiring constant adaptation and oversight.
While AI can enhance trading efficiency and decision-making, its performance is not infallible. Successful trading strategies often integrate AI as part of a broader approach that includes human oversight and risk management to navigate the complexities and uncertainties of financial markets effectively.
Reality 3: Risks and Challenges of AI Trading
Implementing AI in trading introduces a range of risks and challenges that must be carefully managed. One significant challenge is the potential for AI models to perform well in historical data but falter in live market conditions, known as overfitting. This phenomenon arises when AI algorithms are too finely tuned to historical data patterns, leading to poor performance when faced with new or unforeseen market dynamics. Moreover, the inherent complexity of financial markets means that AI models may struggle to adapt to sudden shifts in market sentiment, geopolitical events, or regulatory changes that can significantly impact trading outcomes.
Furthermore, the reliance on AI in trading raises concerns about algorithmic bias and the ethical implications of automated decision-making. Biases embedded in training data or algorithmic design can lead to unfair advantages or unintended consequences in financial markets, potentially amplifying market volatility or exacerbating inequalities.
Navigating these risks requires robust risk management frameworks, ongoing monitoring, and human oversight to ensure AI-driven trading strategies remain adaptive, compliant with regulations, and aligned with broader market objectives. Effective integration of AI involves understanding its limitations and augmenting its capabilities with human judgment to mitigate risks and enhance overall performance in dynamic market environments.
Leave a Reply